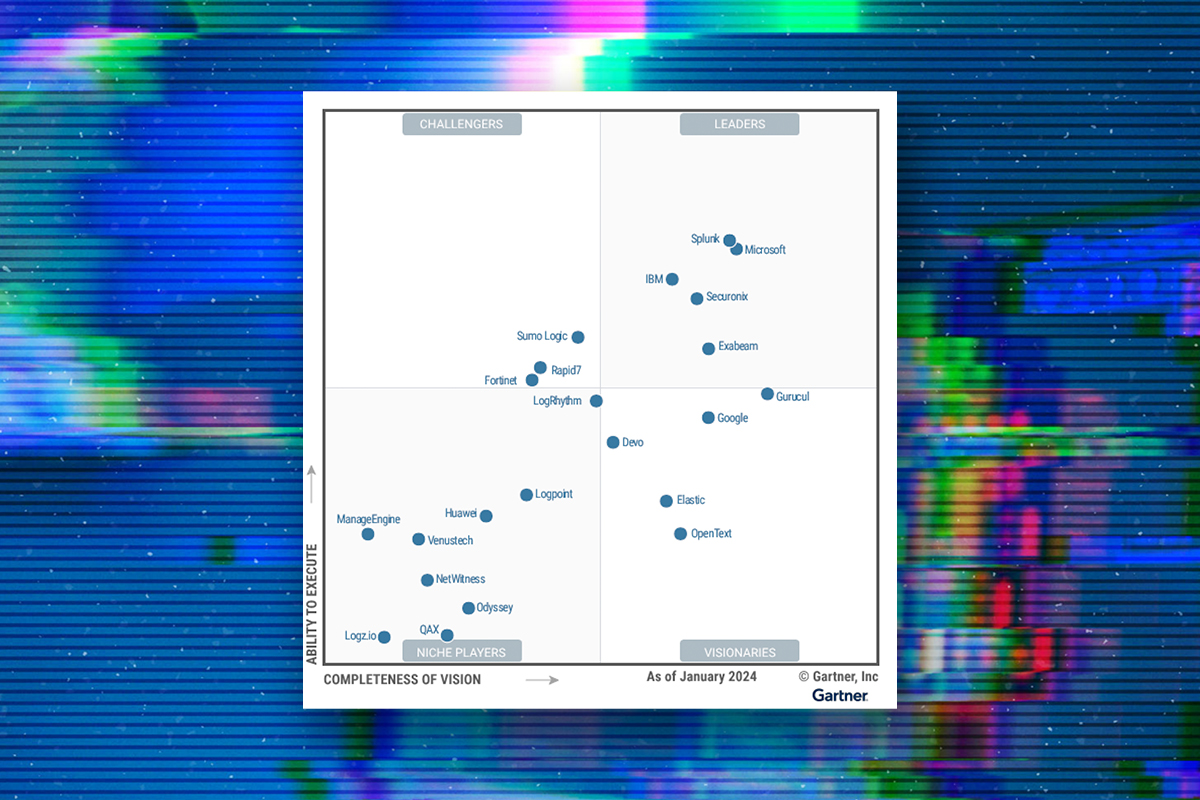
2024 Gartner Magic Quadrant for SIEM
Devo was recognized as a Visionary in the Gartner® Magic Quadrant™ for SIEM based on our Ability to Execute and Completeness of Vision.
Security analytics is the art of combining process, forensics, technology, and skill to detect and react against cyber-threats. It deploys advanced data collection, analysis, and monitoring tools to detect malicious activity and malware that could threaten an organization’s infrastructure.
Security analytics tools detect threats by using a trifecta of data science, AI, and deep learning algorithms on environmental data. It can also combine these big data capabilities with threat intelligence to help detect, analyze, and mitigate insider threats.
This article aims to cover the main features and benefits of security analytics, what it is, and how it can help protect your organization. Traditionally, cybersecurity has taken the “classic approach” where detection is passive, relying on pre-established playbooks and detection rules that only detect well-known threats instead of utilizing multiple data sources. In the modern era, we are taking a more analytical and proactive approach, where data is gathered from multiple sources and analyzed for suspicious behavior with artificial intelligence and machine learning. In the table below we have highlighted these two key methods and their differences.
Classic security tools | Security analytics solutions |
---|---|
Detection based on rules and well-known signatures and uses queries to obtain specific data | Detection based on deviations in pattern learned from multiple data sources |
Approach based on a single event or repeated event | Approach based on user and entity behavior analysis (UEBA) and correlation across dissimilar data sources that finds threats without manual threat-hunting |
Siloed data across applications that needs to be analyzed independently | Approach where data is correlated across unlinked sources for the purpose of detection |
Unidimensional analysis | Multidimensional analysis using machine learning and artificial intelligence to detect threats and deviations from the baseline and moving towards predictive analysis |
Can generate false positives and requires human analysis to eliminate false alerts | Uses advanced techniques to raise attention to actual threats focused on automating tasks |
Requires identification, protection, detection, response and recovery to be independent functions | Allows for identification, protection, detection, response and recovery to be managed holistically from a centralized platform |
As security threats evolve, security standards and frameworks, such as NIST Cybersecurity Framework, have also evolved to counter them. This framework defines five major functions of an efficient cybersecurity strategy:
NIST Cybersecurity Framework main functions (NIST.gov)
When security professionals are able to detect threats at an early stage, they can counter-attack more quickly to prevent network infiltration, infrastructure compromise and data loss.
Example of the types of data ingested into a security analytics solutions
Security analytics solutions thrive on data and the more data points they have the better they function. Data is aggregated and then analyzed for suspicious patterns and behaviors. Sources and types of analytical data include:
These sources all generate logs in various formats that can be ingested by an analytics solution. By combining and correlating multiple data feeds, organizations can now work with a single dataset or “source of truth”. This allows security professionals to centrally apply appropriate algorithms and create rapid searches that can identify early indicators of attack. Remarkably, machine learning technologies can even be used to perform threat and data analysis in near real-time.
Due to the diversity of data sources, security specialists typically use a platform that can gather and analyze data centrally, rather than examining logs in situ on individual systems. Centralized log management improves the efficiency of viewing and analyzing normalized logs from varied sources across systems.
Security analytic processes are generally more successful and easier to implement when data sources are graded for both risk and value. This allows the selection of protective technologies and processes to be based on the resource itself.
Security analytic components
A common challenge for many organizations is managing the security risks associated with multi-cloud and hybrid-cloud environments. By using a security analytics approach, the lack of infrastructure visibility and the difficulty of managing complex data often associated with the cloud can be overcome. For example:
Security analytics allows for a more proactive security posture, improved visibility across systems, and improved incident management by detecting threats earlier, aggregating data from multiple sources, and making patterns emerge. More than that, security analytics also contributes to maintaining regulatory compliance with government and industry regulations, such as HIPAA, PCI-DSS and GDPR. It does so by monitoring access, user behavior and authentication, allowing for better detection of non-compliance. Security analytics also provides greater insight into security incidents by providing forensic, type and origin-of-attack information which helps prevent similar incidents in the future.
Security analytics provides many versatile capabilities that range from improving network visibility to employee monitoring and threat detection. Due to their flexibility, security analytics tools have many use cases – here are some examples:
Applying security analytics to strengthen your security program requires investment in tools, processes, and people. Each analytics technology is quite different from the other, which leads to a requirement for good training and staffing. Without appropriate ability or experience, subsequent misconfiguration or misuse of these tools could result in false alerts and corrective efforts that nullify their benefit.
A good starting point is to build upon any pre-existing capability or experience in your organization. As a recommendation, you should:
As an example, your goal may be to detect insider threats to the organization. Data exfiltration might be identified as a gap, so you should search for solutions that differentiate between data leak traffic and normal traffic. This way you’re able to maximize the potential of Security Analytics through the correlation of data flow logs with existing data log points within the platform.
Some examples of popular security analytics solutions that you may wish to research include:
Defining the selection criteria for a solution that meets all of your needs is not always easy. If in doubt, bare the following tips in mind:
As attack surfaces increase and threat environments become more complex, organizations face new obstacles to preserving their safety, upholding their security and preventing cyber incidents. By correctly aggregating, correlating, and analyzing data, security analytics can act as a bulldozer to these obstacles and provide a level of threat visibility and defense not previously available.