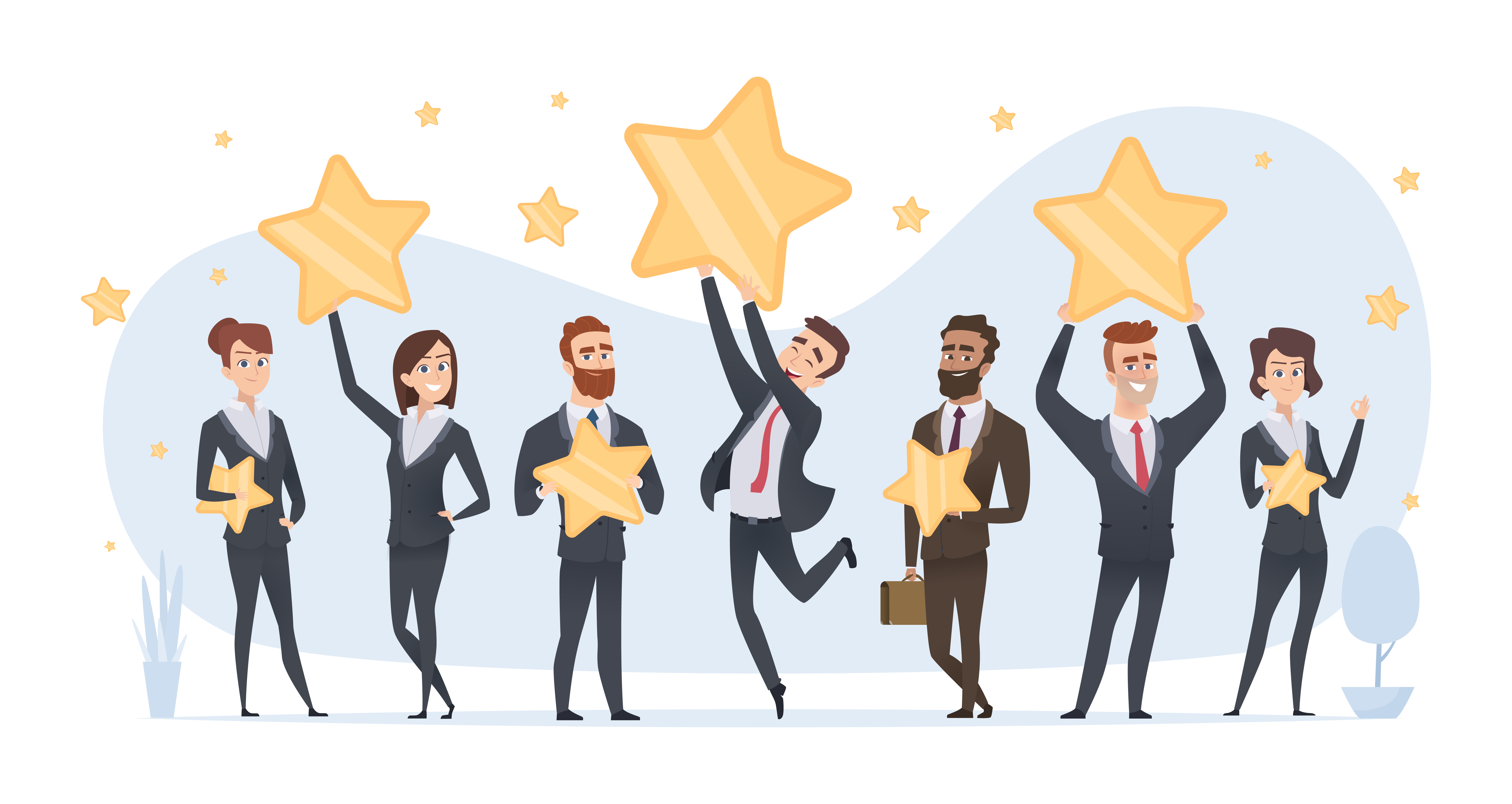
With the rapid expansion in both scale and variety of technologies in modern business systems, there comes a need to further secure those technologies to prevent nefarious actors from causing havoc. The expanding data landscape creates a much larger attack surface for bad actors to exploit, and as a result leaves many organizations at risk from theft, fraud or other undesirable behavior.
As we react to these attacks and attempt to secure our technologies, the attacks become more and more sophisticated, requiring an ever-expanding and changing set of techniques to sustain protection. These unorthodox attack methods now demand faster detection and response, requiring a larger amount of data points in order to not only identify them, but to be proactive in their detection and not reactive to the damage.
User and Entity Behavior Analytics (UEBA) is a key element of the solution to the problem, and when combined with other components of a proper security program can lead to a greater degree of protection, providing the capability to proactively detect and respond to sophisticated threats. UEBA works by employing machine learning and other analytic techniques to identify and correlate anomalous and dangerous behaviors based on the data collected from users and entities throughout an organization’s network. By analyzing this data, a behavior baseline is developed and tuned. This is then used to uncover deviations from normal events, allowing one to bubble up interesting cases that may need to be looked at while tuning down the noise in the environment.
UEBA is not a new concept, and was first coined by Gartner in 2015. So why talk about UEBA now? The vast majority of products in the market supporting UEBA have failed to achieve the full vision and scope as defined by Gartner due to the inability to scale machine learning (ML) models across broad data sources.
With the explosion of data from any number of devices, it has become more and more difficult for solutions to reach the appropriate level of scalability to apply UEBA against large data sets. Many UEBA products have dealt with this by limiting the scope of the data in which they can operate, which greatly reduces the efficacy of the ML models. Ultimately this results in not achieving the mission of UEBA — which is to augment analysts and enable them to spend their time looking at prioritized cases instead of wasting time investigating cases not relevant to actual issues.
Rather than focus purely on the analytics, dashboards, and models, Devo chose to focus first on the data needed to properly supply those models. The result is a highly scalable, performant data system that enables these models to run more efficiently, enabling analysis of trends and patterns across more data types and at larger volumes, while still maintaining a level of performance that is unparalleled.
This allows a modern SOC to become proactive in threat reduction, and creates the opportunity to detect and respond to attacks more frequently, when they are actually being conducted, instead of dealing with the ramifications after the fact. Since the models work across a wide array of data types, it allows for greater visibility than traditional UEBA approaches from other vendors.
Devo Behavior Analytics, provides a solution for UEBA that goes beyond legacy approaches. Devo has taken our highly-scalable and performance security analytics platform and has extended its capabilities with a modern, cloud-native solution to UEBA.
Contact us or reach out to your sales representative or customer success manager to learn more about Devo Behavior Analytics.